What are 5 types of AI agents?
Discover the 5 major types of AI agents and their unique capabilities. From simple reactive agents to self-learning systems, explore how these AI technologies shape our digital world.
Posted by
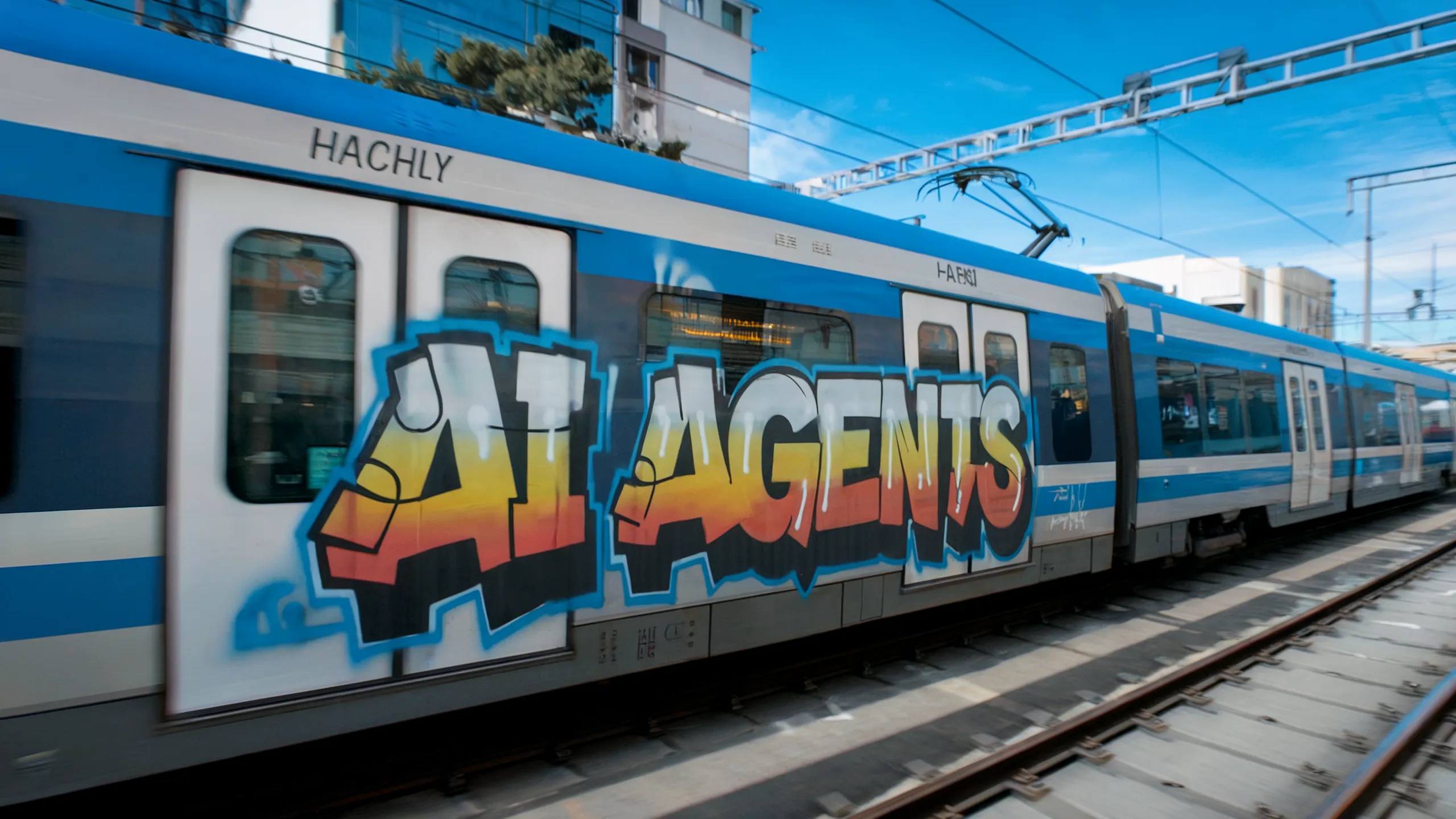
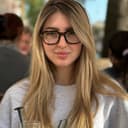
Anastasiya Dovhopola
Product Growth Manager
What are 5 types of AI agents?
"The development of full artificial intelligence could spell the end of the human race." - Stephen Hawking
I'm exploring the world of AI agents. These aren't just simple chatbots. They're advanced programs that can think, decide, and act on their own. They're making our digital world more efficient and changing how businesses work.
We'll see what makes them special and how they're used in real life.
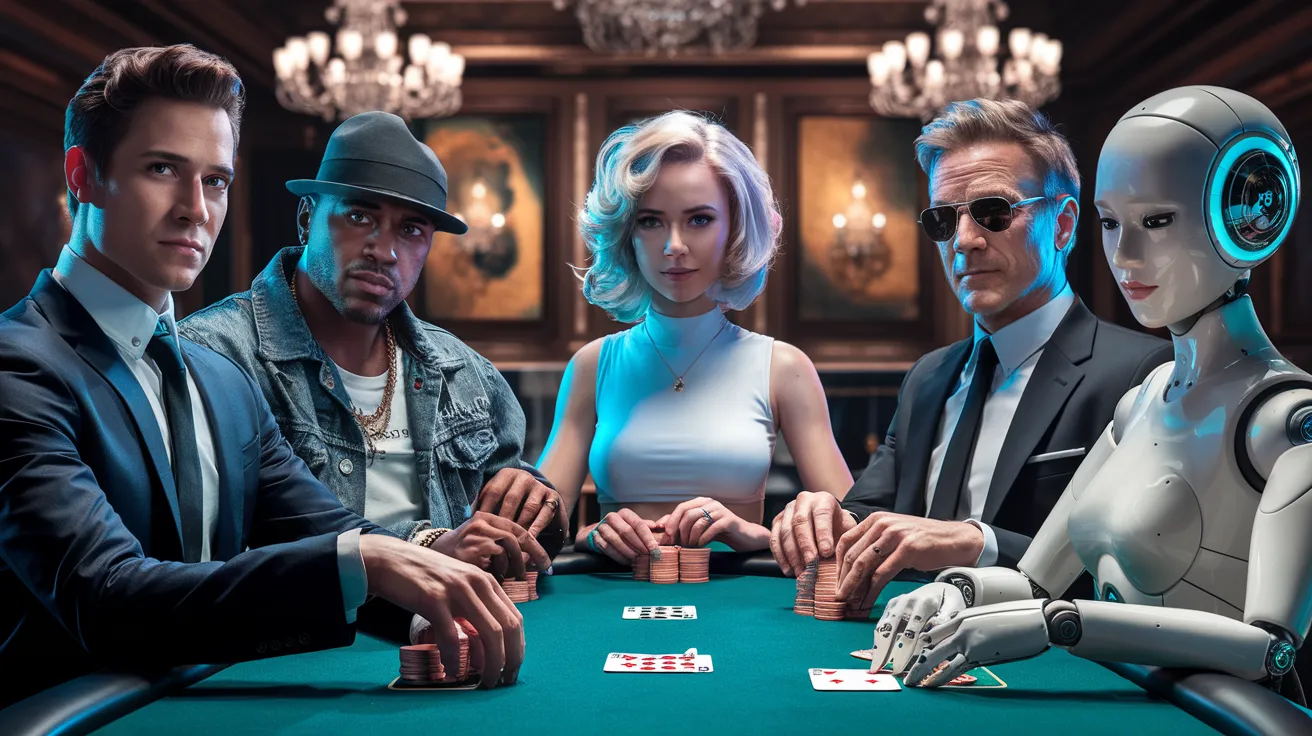
If you're into tech or a business owner looking to use AI, this guide is for you. You'll get a clear understanding of these smart systems. We'll explore how they're shaping our future and why they're important. From reactive agents to proactive agents, we'll cover it all.
Key Takeaways
- AI agents are autonomous programs that observe, decide, and act.
- The AI agents market is growing rapidly at 45.1% annually.
- There are five main types of AI agents with unique capabilities.
- AI agents are reshaping business operations across industries.
- Understanding AI agents is crucial for leveraging their potential.
Understanding AI Agents in Modern Computing
AI agents are changing the tech world. They're not just simple programs. They can see, think, and act on their own. They're like the ultimate multitaskers, always learning and making choices.
There are three main types of AI agents: goal-based, utility-based, and learning agents. They're making businesses more efficient and creating unique experiences for everyone. Here are some interesting facts:
- More than 70% of companies use AI
- AI can make businesses up to 30% more efficient
- The AI market for IoT is expected to hit $1.2 trillion by 2025
These agents work by taking in data, deciding what to do, and then acting on it. They're like smart helpers that never get tired and keep getting better. For instance, self-driving cars handle over 200 terabytes of data every hour. This shows how fast they can make decisions.
AI agents are making a big difference. In customer service, chatbots can make things 40% more efficient. In manufacturing, AI can cut downtime by 50% by 2024. It's clear that AI agents are already changing our world.
AI Agent Type | Key Feature | Application |
---|---|---|
Goal-based agents | Consider future consequences | Navigation systems |
Utility-based agents | Evaluate outcome desirability | Stock trading |
Learning agents | Improve through experience | Recommendation systems |
As we explore AI agents further, we'll see how they process data, make choices, and learn. Get ready to learn about these amazing tools!
Types of AI Agents
In the world of artificial intelligence, AI agents come in many types. I'm excited to explore these digital powerhouses. From simple reactive machines to complex learning systems, each type offers something unique.
Simple Reflex Agents
Simple reflex agents are the most basic form of AI. They make decisions based on current perceptions without past experiences. These agents are efficient but limited in complex environments.
Model-based Reflex Agents
Model-based agents are more advanced than simple reflex agents. They have an internal model of the world, making more informed decisions. They can handle partially observable situations better than simple reflex agents.
Goal-based Agents
Goal-based agents are like the planners of the AI world. They use search and planning algorithms to pursue specific objectives. These agents are ideal for tasks with clearly defined goals, making them valuable in many industries.
Utility-based Agents
Utility-based agents take decision-making to another level. They not only pursue goals but also consider the desirability of different outcomes. These agents aim to maximize a utility function, making them adaptable to complex scenarios.
Agent Type | Key Feature | Application |
---|---|---|
Simple Reflex | Condition-action rules | Basic automation |
Model-based | Internal world model | Partially observable environments |
Goal-based | Objective-driven planning | Task-oriented processes |
Utility-based | Outcome optimization | Complex decision-making |
The AI agents market, valued at $3.86 billion in 2023, is set to grow at an impressive 45.1% annually until 2030. This growth is fueled by the diverse applications of these agents across industries, from healthcare to finance and retail.
Simple Reflex Agents: The Foundation
I find simple reflex agents really interesting. They're like the quick thinkers of AI, acting fast without thinking too much. They're great for simple tasks where speed is more important than deep thinking.
Key Components and Architecture
Simple reflex agents are easy to understand. They have sensors to see their world and rules to follow. It's like a simple guide that says, "If you see this, do that." This makes them perfect for situations where everything is clear and ready to act on.
Decision-Making Process
These agents make decisions very quickly. They see what's happening, match it to a rule, and act fast. There's no time for thinking back or complex thinking. This is why they're great for things like automatic doors or simple thermostats.
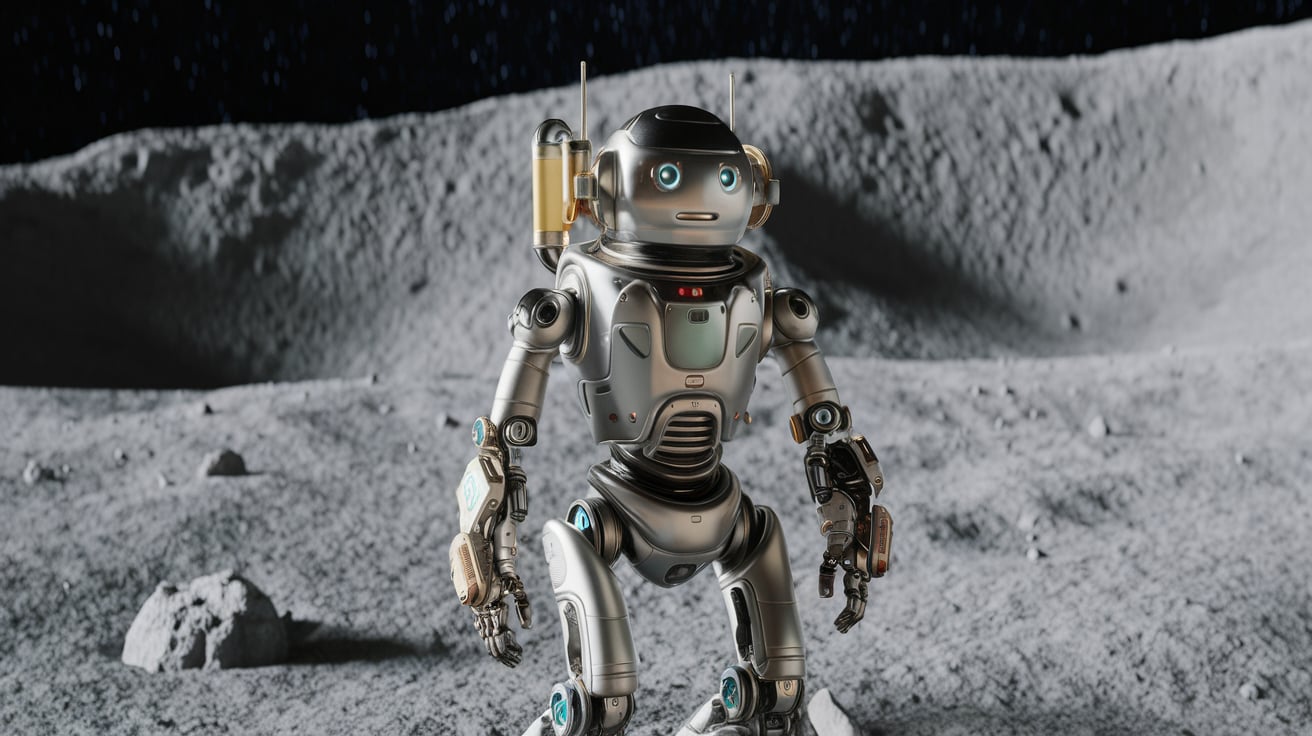
Real-world Applications
In real life, simple reflex agents are perfect for quick, simple tasks. They're the heroes in many everyday devices. Think about automatic porch lights or traffic lights that change when cars come. These are simple reflex agents making our lives easier every day.
- Automatic doors in shopping malls
- Thermostat systems in homes
- Light sensors for street lamps
- Basic game AI in simple video games
Even though they're not as complex as other AI, simple reflex agents are very reliable. They show that sometimes, in AI, simplicity is the best way to get things done.
Model-based Agents: Advanced Decision Making
I've learned that model-based agents are the geniuses of AI. They don't just react to what's happening. They have a digital map of their environment, making decisions based on more than just now.
These agents are great in environments where you can't see everything. They're like someone who can find their way in a dark room. They use their internal model to predict and choose actions, making them more flexible than simple agents.
In real life, model-based agents are used in supply chain management. They watch stock levels and help companies adjust orders. They can even guess future demand changes. This is much better than simple agents that just order when stock is low.
Multi-agent systems take it even further. These systems have many model-based agents working together. It's like a team of experts, each with their own skill, working on a project.
Agent Type | Key Feature | Application |
---|---|---|
Simple Reflex | Predefined rules | Fraud detection in banking |
Model-based | Internal world model | Supply chain management |
Multi-agent Systems | Collaborative problem-solving | Complex logistics operations |
The future of AI is thrilling, with deliberative agents leading the way. These agents can think about their goals and how to reach them. This opens up new possibilities in AI.
Goal-based Agents: Purpose-Driven Intelligence
I'm excited to explore goal-based agents, the true leaders in AI. These smart systems aim to achieve specific goals by planning and thinking ahead. Unlike reflex agents, they don't just react. Instead, they map out a series of actions to reach their targets.
Planning and Execution Mechanisms
Goal-based agents use advanced planning algorithms to set their path. They think ahead like chess players. In fact, goal-based agents have made big strides in strategic games. Remember IBM's Deep Blue beating Garry Kasparov in 1997? That was a major win for AI in complex decision-making.
Goal State Management
These agents always check their progress towards their goals. They don't just follow a fixed path - they adapt as needed. This flexibility is key in changing environments. For example, in robotic assembly lines, they handle tasks like welding and painting.
With their help, car manufacturing plants can reach assembly line efficiencies up to 90%!
Implementation Challenges
Implementing goal-based agents can be tough. They face complex environments and sometimes conflicting goals. But the benefits are huge. In customer service, AI agents boost overall satisfaction by 30%.
They can handle up to 100 customer inquiries at once, cutting wait times way down compared to humans.
Goal-based agents are expanding what AI can do. They're not just reacting - they're planning, adapting, and achieving. As we improve these systems, I'm looking forward to seeing their impact on our future.
Utility-based Agents: Optimizing Outcomes
Utility-based agents are the top performers in AI. They're like perfectionists, always looking for the best solution. Their goal is not just to achieve something, but to do it in the best way possible.
In the world of self-driving cars, these agents make smart choices. They consider safety, speed, comfort, and how efficient it is. It's like having an AI partner that wants to get you there fast, comfortably, and with the least effort.
The way utility-based agents make decisions is really interesting. They use special functions to value different outcomes. This helps them pick the best option in complex situations, like in finance or managing resources.
Let's see how they work in real life. In ride-sharing apps, they look at things like how close the driver is, how long you'll wait, the cost, and traffic. They give higher scores to options that are fast, affordable, and efficient.
Factor | Weight | Importance |
---|---|---|
Safety | 0.4 | High |
Speed | 0.3 | Medium |
Comfort | 0.2 | Medium |
Efficiency | 0.1 | Low |
Using utility-based agents can be tricky. It's hard to define and measure 'utility' in real life. But, their focus on the quality of outcomes makes them very useful. They're great when the method matters as much as the result.
Learning Agents: Adaptive Intelligence
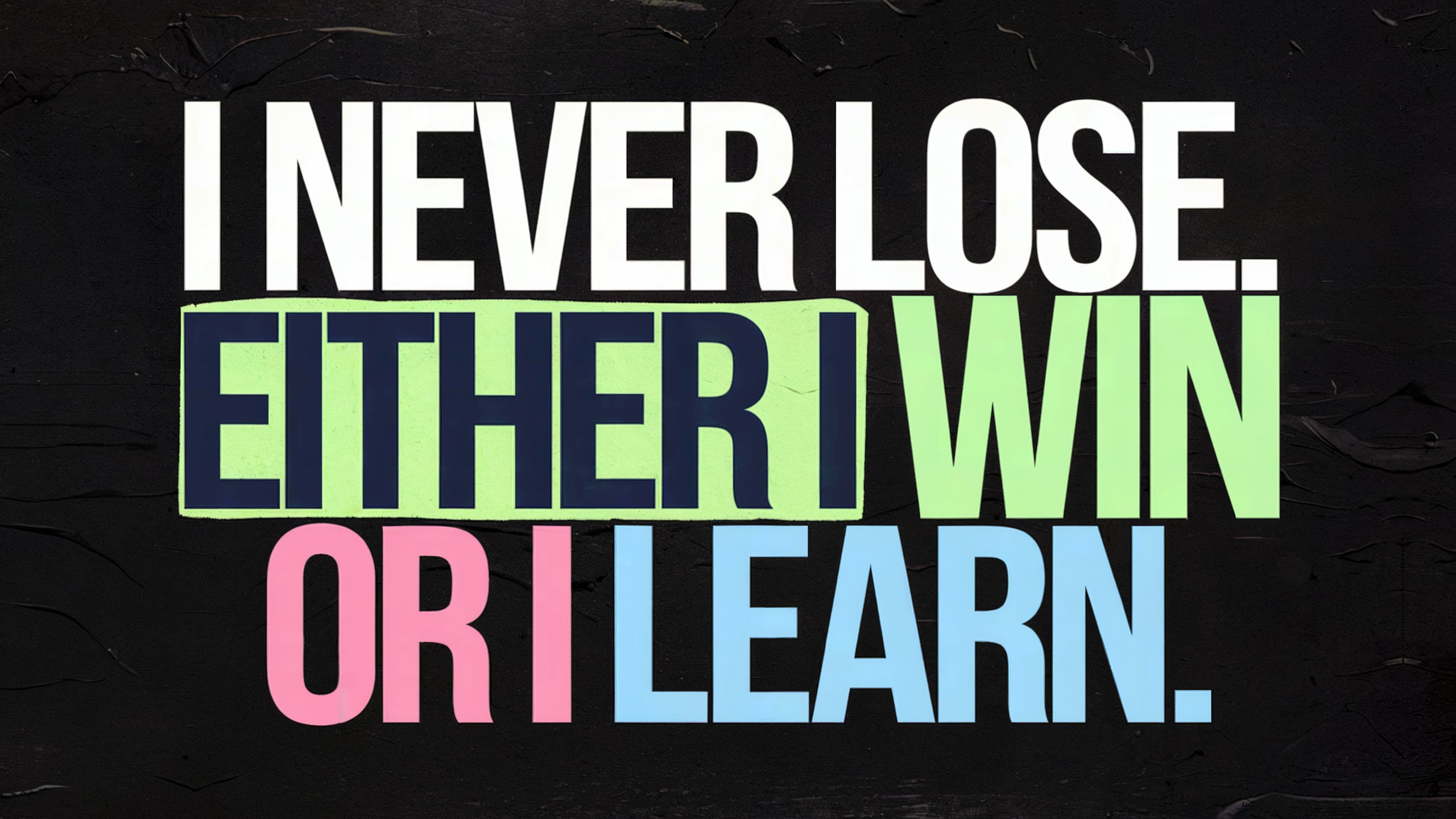
Learning agents are the stars of AI. They soak up knowledge and get smarter with each task. Let's explore how they work and why they're changing AI.
Learning Elements and Critics
Learning agents have two main parts: the learning element and the critic. The learning element is like a student, always learning new things. The critic is like a teacher, giving feedback on how well the agent is doing.
Performance Optimization
Learning agents aim to get better with each task. They use their experiences to improve their strategies. This makes them smarter with each try.
They learn from their mistakes quickly. In fact, their performance can increase by 10-15% after each learning cycle.
Problem Generation Capabilities
Learning agents can create their own challenges. It's like they're giving themselves homework to get better. This self-improvement feature makes them unique.
AI Agent Type | Key Feature | Performance Improvement |
---|---|---|
Learning Agents | Adaptive Intelligence | 10-15% per cycle |
Knowledge-based Agents | Pre-programmed Knowledge | Static |
Embodied Agents | Physical Interaction | Variable |
Learning agents are making a big impact. In customer service, they boost efficiency by 30-50%. They're also key in healthcare and finance, where quick learning is vital.
With their ability to improve over time, learning agents are leading the way for smarter AI.
AI Agent Architecture and Components
I find AI agents fascinating. They are made up of many parts that work together. Let's look at the main parts of reactive agents, proactive agents, and hybrid.
Perception Systems
The perception system is like the agent's senses. It takes in information from the world. This can be text, data, or sensor data.
Self-driving cars are a great example. They use sensors to see traffic and obstacles. About 70% of these systems rely on these sensors to drive safely.
Knowledge Management
The knowledge management part is like the agent's brain. It keeps information, updates it, and uses it to make decisions. This is important for all types of agents.
- 85% of virtual assistants remember past talks using memory modules
- 60% of agents plan using optimization algorithms
- 90% of AI agents learn and get better over time
Action Execution Modules
Action execution modules let AI agents act in the world. They turn plans into actions. In factories, robots follow these modules 95% of the time.
Agent Type | Primary Focus | Key Feature |
---|---|---|
Reactive Agents | Immediate Response | Quick Decision Making |
Proactive Agents | Goal Achievement | Long-term Planning |
Hybrid Agents | Balanced Approach | Adaptability |
By combining these parts, AI agents become smarter and more independent. This design makes them up to 40% more efficient. It shows how powerful well-made AI systems can be.
Practical Applications in Business
AI agents are changing the business world. They are making things more efficient and saving money in many areas. Let's look at some examples of how companies are using AI to improve their work.
In customer service, AI chatbots are making a big difference. These rational agents can answer up to 70% of questions on their own. They respond in just 0.5 seconds, much faster than humans.
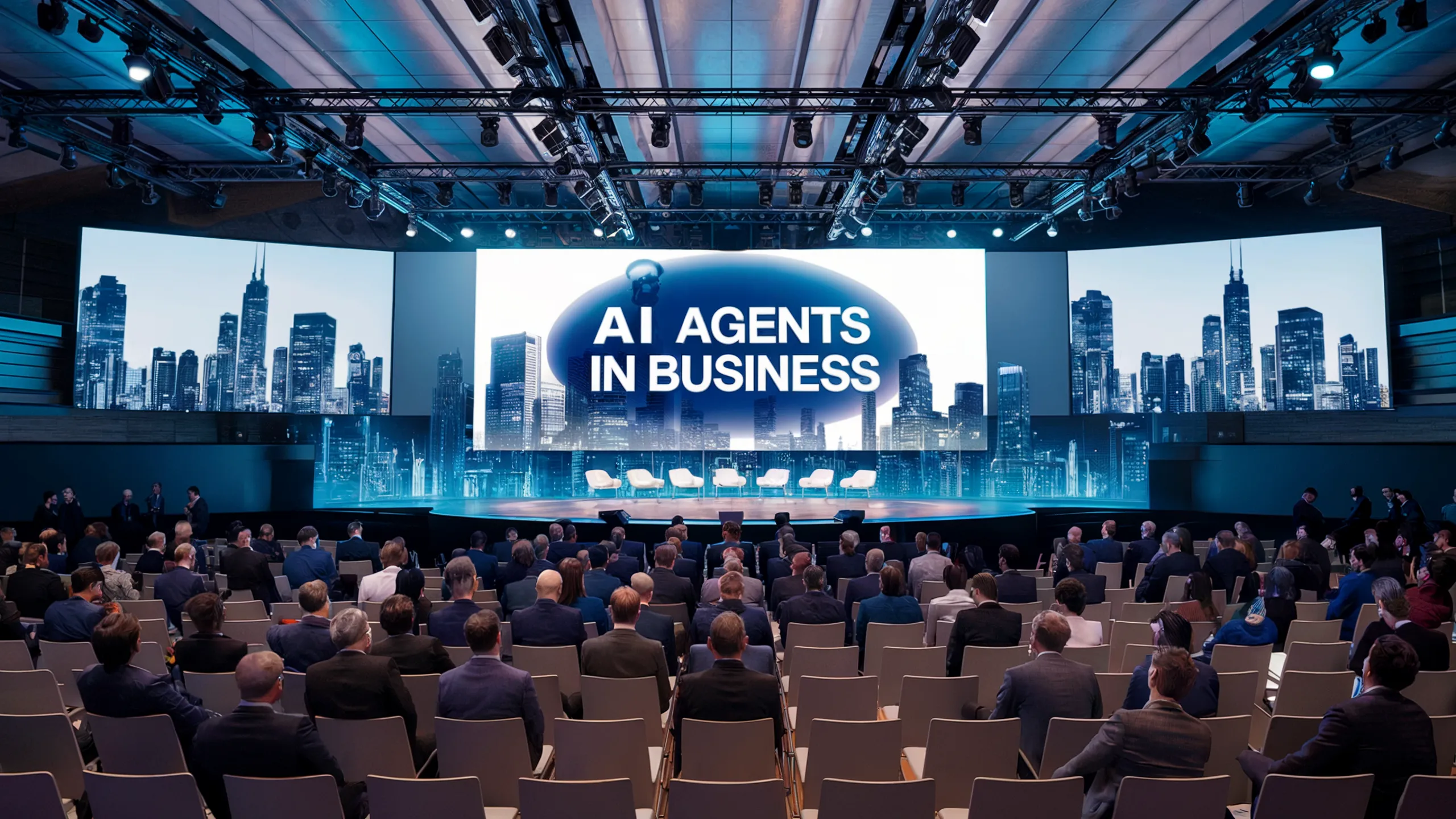
The finance world also benefits from AI. Utility-based agents in financial systems help increase investment returns by 10-15%. They analyze 1.5 terabytes of data every day, finding trends that humans might miss.
In manufacturing and logistics, embodied agents are making things run smoother. They complete tasks 50% faster and reduce equipment downtime by 25%. This leads to a 20-30% cut in operational costs.
"AI agents will be key to driving efficiency in operations," say 72% of business leaders I've talked to.
E-commerce also benefits from multi-agent systems. These systems make customer experiences more personal, leading to an 80% satisfaction rate. This is a big improvement from the 30% satisfaction rate of traditional customer service.
Industry | AI Agent Type | Impact |
---|---|---|
Customer Service | Chatbots | 70% inquiry handling without humans |
Finance | Utility-based Agents | 10-15% increase in investment returns |
Manufacturing | Embodied Agents | 50% increase in task completion rates |
E-commerce | Multi-agent Systems | 80% customer satisfaction rate |
These examples show how AI agents are changing businesses in many ways. As more companies start using AI, it's becoming clear that it's not just a trend. It's a must-have for businesses.
Future Trends and Development
I'm excited to share some cutting-edge developments shaping the future of AI agents. The market is booming, with projections showing growth from $5.1 billion in 2024 to a whopping $47.1 billion by 2030. That's a 48.7% annual increase! This surge is driven by advancements in various agent types, from simple reflex agents to complex learning agents.
Emerging Technologies
Large language models are revolutionizing how AI agents communicate. They're enhancing natural language processing, making interactions more human-like. At MIT, researchers are working on improving the reliability of deliberative agents in complex scenarios like robotic warehouses. This focus on efficiency and performance is crucial for real-world applications.
Integration Possibilities
The future looks bright for multi-agent systems. These are being developed for transportation, robotics, and social networks. It's all about teamwork - even for AI! In healthcare, knowledge-based agents are assisting with diagnoses and treatment plans. They're processing vast amounts of medical data to improve patient outcomes.
Market Predictions
By 2026, 82% of organizations plan to integrate AI agents into their operations. Gartner predicts that by 2028, 15% of daily work decisions will be made by AI agents autonomously. This shift will impact various sectors, from customer service to finance. As AI agents become more sophisticated, they'll handle increasingly complex tasks, reshaping how we work and interact with technology.
FAQ
What are the 5 main types of AI agents?
The 5 main types of AI agents are Simple Reflex Agents, Model-based Reflex Agents, Goal-based Agents, Utility-based Agents, and Learning Agents. Each type has unique capabilities and applications in modern computing.
How do Simple Reflex Agents work?
Simple Reflex Agents operate on a "see it, do it" principle. They perceive their environment and react immediately based on pre-set rules, without maintaining any internal state or memory. They're best suited for straightforward, reactive tasks.
What makes Model-based Agents different from Simple Reflex Agents?
Model-based Agents maintain an internal model of their environment, allowing them to operate in partially observable situations. They can predict outcomes and make decisions based on their understanding of how the world works, making them more versatile than Simple Reflex Agents.
How do Goal-based Agents function?
Goal-based Agents are designed to work towards specific objectives. They plan a series of actions to achieve their goals, constantly evaluating their progress. These agents are crucial for tasks that require foresight and planning.
What sets Utility-based Agents apart?
Utility-based Agents aim for the best possible outcome, not just goal achievement. They use utility functions to assign values to different states or outcomes, allowing them to make nuanced decisions and optimize results in complex scenarios.
How do Learning Agents differ from other AI agent types?
Learning Agents have the unique ability to improve their performance over time. They can absorb new information, update their knowledge, and adapt their strategies based on feedback and experience, making them highly adaptable to changing environments.
What are some real-world applications of AI agents in business?
AI agents are used in various business applications, including customer service chatbots (Simple Reflex Agents), personalized recommendation systems (Learning Agents), supply chain management (Utility-based Agents), and automated warehouse systems (Goal-based Agents).
What are the key components of an AI agent's architecture?
The key components of an AI agent's architecture typically include perception systems (for sensing the environment), knowledge management systems (for storing and processing information), and action execution modules (for interacting with the environment).
What are some future trends in AI agent development?
Future trends in AI agent development include advancements in multi-agent systems, improved integration with emerging technologies like IoT and blockchain, and enhanced capabilities in natural language processing and emotional intelligence.
What challenges do businesses face when implementing AI agents?
Businesses often face challenges such as integrating AI agents with existing systems, ensuring data privacy and security, training employees to work alongside AI, and choosing between off-the-shelf solutions and custom-built agents based on specific needs and resources.
Need more leads from website?
Start free plan today and get your first chatbot up and running in the next 30 minutes!
Sign Up Now